Understanding Bounding Boxes: A Comprehensive Guide
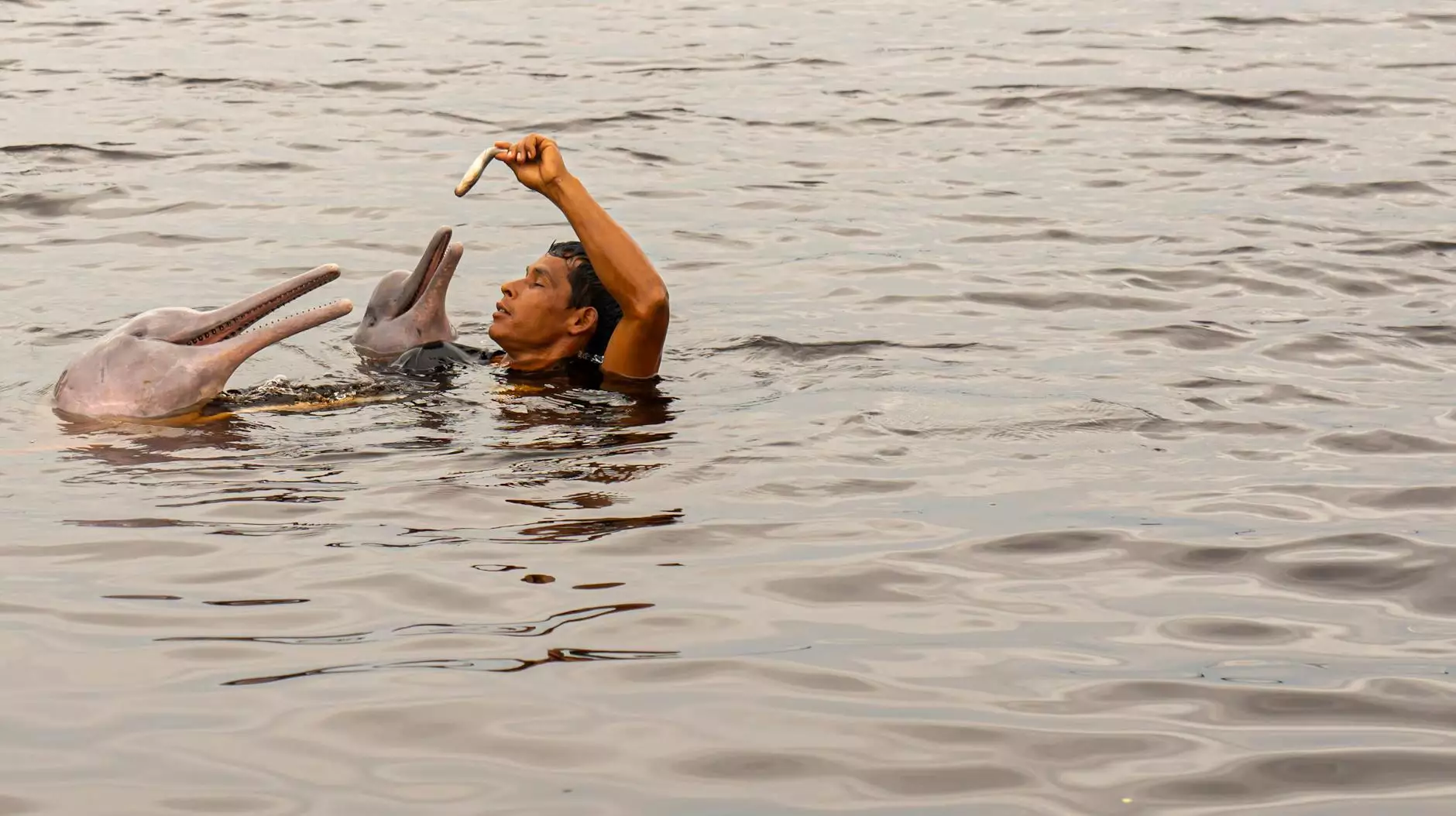
In the realm of computer vision and images, one term that frequently surfaces is bounding box. Whether you are working with data annotation tools or diving into the intricacies of machine learning algorithms, understanding the concept of a bounding box is crucial. This article will explore the bounding box, its applications, significance in various fields, and how it enhances business processes.
What is a Bounding Box?
A bounding box is defined as a rectangle that encloses a specific object within an image. It is characterized by two key aspects: its position (defined by coordinates) and its dimensions (width and height). This fundamental tool helps in identifying and categorizing objects featured in images.
The Importance of Bounding Boxes in Data Annotation
Data annotation is a pivotal process in creating datasets that train machine learning models. The role of the bounding box is particularly significant in this context:
- Object Detection: Bounding boxes are used to train models to identify and locate objects within images. For instance, in a dataset of cars and pedestrians, bounding boxes help distinguish these entities.
- Segmentation: Although bounding boxes provide a general area, they can be instrumental in training more complex segmentation models that require precise object delineation.
- Data Preprocessing: Annotated data is essential for supervised learning. Bounding boxes assist in preprocessing by providing structured information regarding the presence of specific items in the data.
Applications of Bounding Boxes
The usage of bounding boxes extends across various domains. Here are several key applications:
1. Autonomous Vehicles
One of the most cited applications of bounding boxes is seen in the technology behind autonomous vehicles. In order for vehicles to navigate safely and efficiently, they must recognize their surroundings:
- Bounding boxes help detect pedestrians, traffic signals, other vehicles, and obstacles, ensuring that the car can make informed driving decisions.
- Real-time image processing relies heavily on bounding boxes to minimize risks during navigation.
2. Facial Recognition Technology
Facial recognition systems utilize bounding boxes to identify and classify human faces:
- Algorithms first generate bounding boxes around a detected face and then analyze various features to recognize the individual.
- This approach is pivotal in security systems and user recognition applications.
3. Retail and Inventory Management
Modern retail is increasingly reliant on automation, with bounding boxes playing a key role:
- Systems equipped with image recognition capabilities use bounding boxes to monitor stock levels and identify product availability.
- By automating inventory checks, businesses save time and enhance operational efficiency.
How Businesses Leverage Bounding Boxes
In corporate environments, particularly for businesses like KeyLabs.ai, which focus on data annotation platforms, bounding boxes serve as a core method for enhancing productivity and accuracy:
- Improved Accuracy: Accurate data annotation using bounding boxes ensures that machine learning models perform with higher precision, which translates into better end-user experiences.
- Cost Efficiency: Automating the process of data labeling using advanced bounding box techniques can significantly reduce operational costs, allowing for faster project completions.
- Scalability: Businesses can easily scale their data annotation operations by adopting tools that emphasize effective use of bounding boxes.
Implementing Bounding Boxes in Your Projects
For organizations looking to implement bounding boxes in their workflow, here are the steps to consider:
- Choose the Right Tool: Select a data annotation tool that supports bounding box functionality. KeyLabs.ai provides intuitive solutions tailored for various industries.
- Define Your Objectives: Understand what you aim to achieve with your bounding box annotations, whether it is for training a model, improving an app, or enhancing security measures.
- Annotate Your Data: Begin the data annotation process. Ensure that the bounding boxes accurately encompass the target objects for optimal results.
- Train Your Model: Utilize your annotated data sets to train your machine learning models, enabling them to learn from the defined bounding boxes.
- Continuous Feedback Loop: Implement a system for reviewing and revising annotations to improve accuracy over time, ensuring the ongoing effectiveness of your machine learning models.
The Future of Bounding Boxes in AI
The evolution of artificial intelligence and computer vision is paving the way for more sophisticated methods in object detection that might move beyond traditional bounding boxes:
- 3D Bounding Boxes: As technology advances, so does the possibility of implementing three-dimensional bounding boxes, allowing for complex object recognition in various conditions.
- Integration with Other Tools: Future platforms may integrate bounding box techniques with other forms of analysis, such as semantic segmentation, to enhance understanding and process efficiency.
Conclusion
In summary, the concept of bounding boxes is an indispensable component in the fields of data annotation and computer vision. For businesses, especially those in technology and retail sectors like KeyLabs.ai, mastering the use of bounding boxes can lead to improved product offerings, soaring efficiency, and enhanced customer satisfaction. As technology continues to evolve, staying ahead of developments in bounding box applications will be crucial to maintaining competitive advantages in the market.